Unlock the Potential of Image Dataset for Object Detection
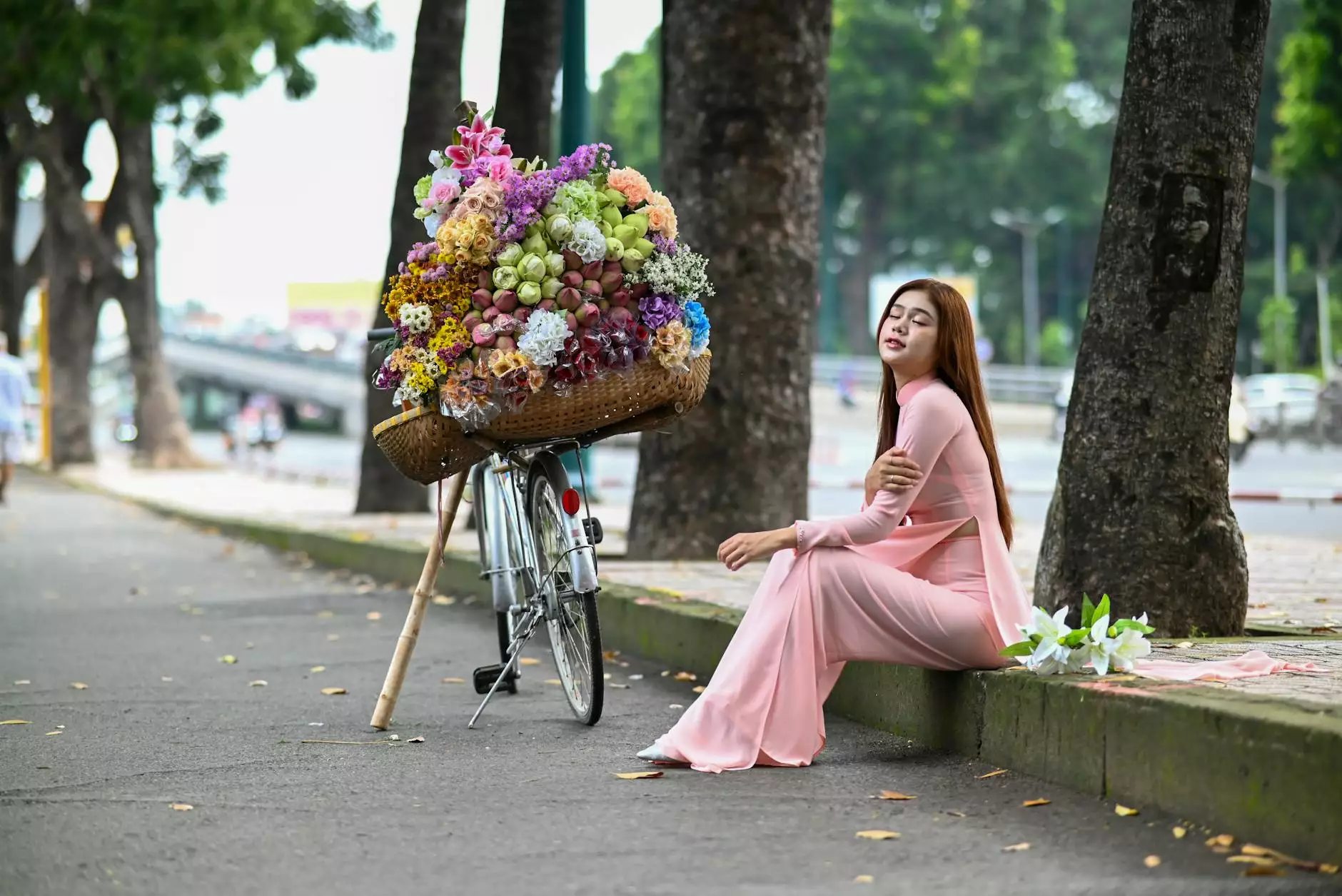
The age of artificial intelligence is upon us, transforming industries and redefining operational capabilities. At the heart of this revolution lies one crucial component: data. Among the vast types of data used, the image dataset for object detection plays a pivotal role. As organizations seek to harness the power of machine learning and computer vision, understanding these datasets becomes imperative. In this comprehensive article, we will delve deep into why image datasets are essential for object detection, how they are created, and how KeyLabs.ai's leading data annotation tools can streamline your data management efforts.
The Significance of Image Datasets in Object Detection
Object detection is a vital capability that allows systems to identify and classify objects within an image rapidly. This functionality is utilized across numerous applications, from security surveillance and autonomous vehicles to medical imaging and retail analytics. The performance of object detection algorithms heavily relies on the quality and size of the datasets used for training. Here are a few reasons why image datasets are crucial:
- Training and Accuracy: High-quality datasets ensure models learn robust features necessary for accurate predictions. The more diverse and representative the dataset, the better the model's generalization ability.
- Benchmarking Models: Datasets often come with established benchmarks that help compare different models in terms of accuracy, speed, and efficiency.
- Real-World Application: Well-annotated datasets simulate real-world scenarios, enabling the deployment of object detection systems that perform well in practical situations.
Creating an Effective Image Dataset for Object Detection
Creating an *image dataset for object detection* that supports machine learning models involves several steps:
1. Data Collection
The first step in assembling an image dataset is data collection. Organizations can source images from various channels, including:
- Publicly available datasets
- Web scraping techniques
- In-house photography or video recordings
2. Data Annotation
Once the images are collected, they must be annotated appropriately. Data annotation involves labeling the objects in the images with bounding boxes, polygons, or masks, depending on the complexity of the object detection task. Techniques used include:
- Bounding Box Annotation: Simplest form where a rectangular box surrounds the object.
- Polygon Annotation: Used for more complex shapes where bounding boxes are insufficient.
- Semantic Segmentation: Each pixel in the image is labeled, which is useful for detailed segmentation tasks.
3. Data Augmentation
To enhance the dataset's richness, data augmentation techniques can be applied. These methods alter existing images to create variations, such as:
- Flipping images
- Rotating
- Adjusting brightness and contrast
4. Validation and Quality Assurance
Ensuring the integrity and quality of the dataset is crucial. Regular reviews and validation processes should be implemented to maintain high annotation standards. This can include random sampling of images for quality checks and user feedback loops.
Discover KeyLabs.ai: Streamlining Your Data Annotation Process
KeyLabs.ai offers a cutting-edge data annotation tool designed to simplify the creation of image datasets. Our platform provides:
- User-Friendly Interface: Designed for ease of use, allowing annotators to label data quickly and accurately.
- Collaboration Features: Teams can work together seamlessly across different projects, ensuring consistency and quality.
- Advanced Annotation Tools: Multiple annotation methods (bounding boxes, polygons, etc.) to suit various project needs.
- Quality Control Mechanisms: Automated checks help maintain annotation standards and improve dataset reliability.
The Future of Object Detection
As technology advances, so does the complexity of the object detection tasks. The future will see enhanced algorithms that require even more sophisticated image datasets. This demands a shift in how datasets are generated and annotated. With the rise of deep learning, models can effectively learn from high-dimensional data; however, they still require extensive datasets for effective training.
Trends Shaping the Direction of Image Datasets
Several emerging trends are influencing the development of image datasets within the domain of object detection:
- Open Datasets: The sharing of labeled datasets among researchers accelerates innovation and enables faster model training.
- Automated Annotation: Tools powered by machine learning can annotate images by learning from already labeled data, ensuring faster preprocessing.
- Ethical Data Use: As issues regarding data privacy and ethical considerations become prominent, the call for transparent and fair practices in data collection and usage will grow.
Conclusion: Empowering Your Business Through Data
In conclusion, a robust and well-annotated image dataset for object detection is a vital asset for businesses looking to leverage AI and machine learning effectively. By integrating solutions like KeyLabs.ai's data annotation platform, organizations can enhance their data preparation processes, leading to better-performing models and, ultimately, increased operational efficiency.
As we continue to embrace the power of AI, the ability to correctly identify and utilize quality image datasets will separate successful companies from the rest. Unlock the potential of your data today and watch your business transform into a data-driven powerhouse.